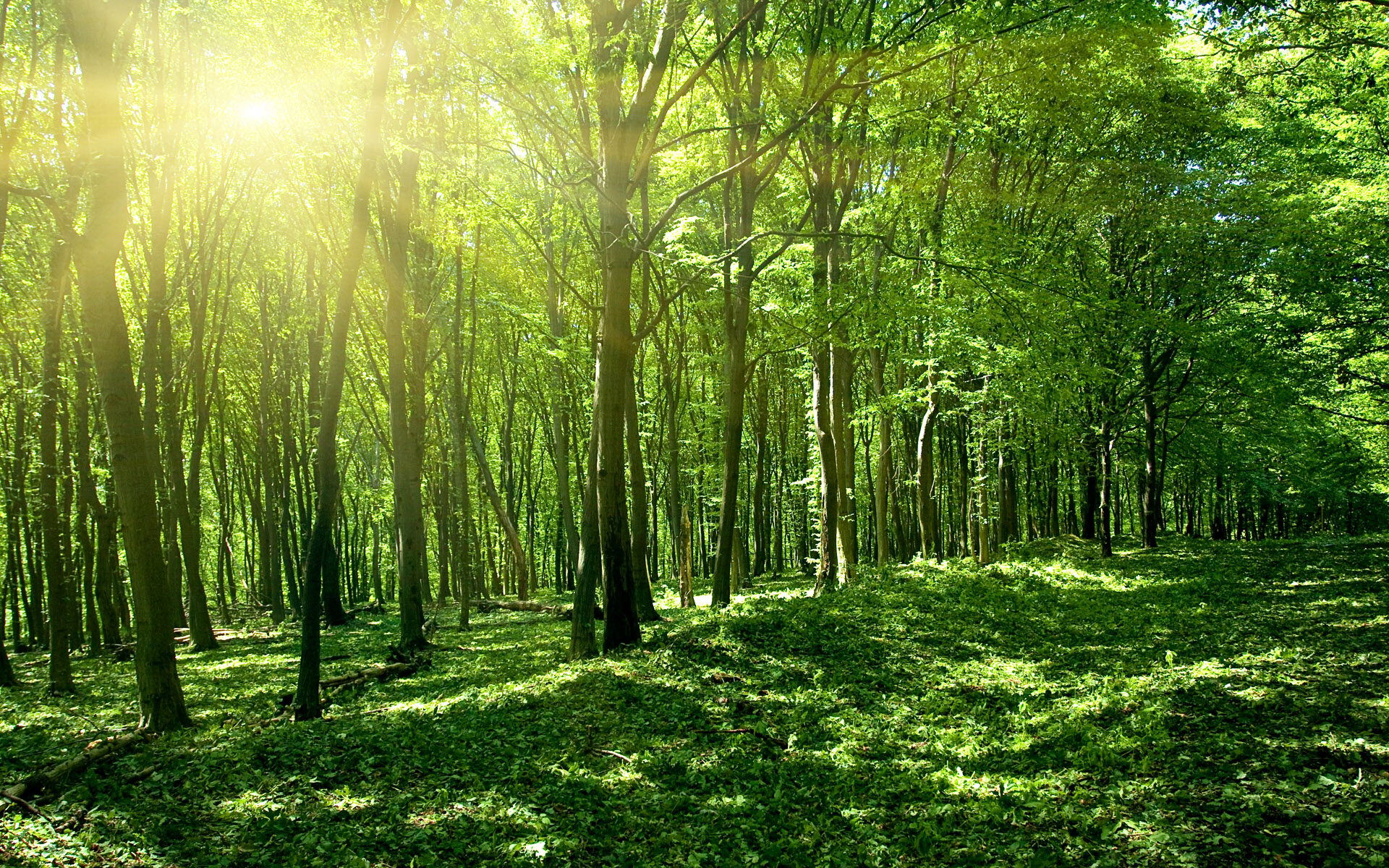
深度 Q 网络(Deep - Q - Network) 介绍
在 Q-learning 算法中,当状态和动作空间是离散且维数不高时,可使用 Q-table 储存每个状态动作对的 Q 值,然后通过贝尔曼方差迭代求得每个状态动作对收敛的 Q 值,然后选择最优的动作当做策略。但是而当状态和动作空间是高维连续时,比如(游戏的状态动作对数目就很大)使用 Q-table 存储每个状态动作对就显得很不现实。
所以可以将 Q-Table 的更新问题变成一个函数拟合问题,相近的状态得到相近的输出动作。DQN 就是要设计一个神经网络结构,通过函数来拟合 Q 值。
下面引用一下自己写的一篇综述里面的 DQN 训练流程图,贴自己的图,不算侵犯版权吧,哈哈。知网上可以下载到这篇文章:http://kns.cnki.net/kcms/detail/detail.aspx?dbcode=CJFD&&filename=JSJX201801001。当时3个月大概看了百余篇DRL方向的论文,才写出来的,哈哈。
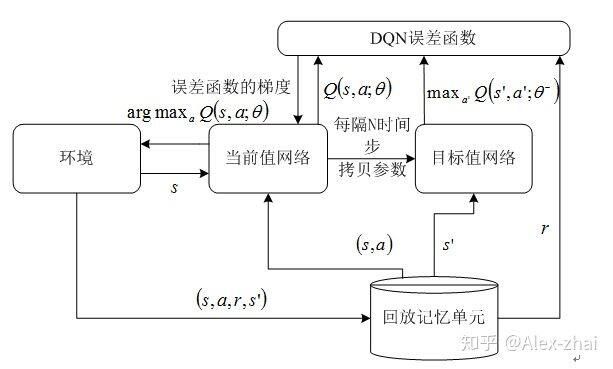
DQN 的亮点:
通过 experience replay(经验池)的方法来解决相关性及非静态分布问题,在训练深度网络时,通常要求样本之间是相互独立的,所以通过这种随机采样的方式,大大降低了样本之间的关联性,从而提升了算法的稳定性。
使用一个神经网络产生当前 Q 值,使用另外一个神经网络产生 Target Q 值。
DQN 损失函数和参数更新:
损失函数:

其中 yi 表示值函数的优化目标即目标网络的 Q 值:

参数更新的梯度为:

Tensorflow 2.0 实现 DQN
整体的代码是借鉴的莫烦大神,只不过现在用的接口都是 Tensorflow 2.0,所以代码显得很简单,风格很像 keras。
# -*- coding:utf-8 -*-
# Author : zhaijianwei
# Date : 2019/6/19 19:48
import tensorflow as tf
import numpy as np
from tensorflow.python.keras import layers
from tensorflow.python.keras.optimizers import RMSprop
from DQN.maze_env import Maze
class Eval_Model(tf.keras.Model):
def __init__(self, num_actions):
super().__init__('mlp_q_network')
self.layer1 = layers.Dense(10, activation='relu')
self.logits = layers.Dense(num_actions, activation=None)
def call(self, inputs):
x = tf.convert_to_tensor(inputs)
layer1 = self.layer1(x)
logits = self.logits(layer1)
return logits
class Target_Model(tf.keras.Model):
def __init__(self, num_actions):
super().__init__('mlp_q_network_1')
self.layer1 = layers.Dense(10, trainable=False, activation='relu')
self.logits = layers.Dense(num_actions, trainable=False, activation=None)
def call(self, inputs):
x = tf.convert_to_tensor(inputs)
layer1 = self.layer1(x)
logits = self.logits(layer1)
return logits
class DeepQNetwork:
def __init__(self, n_actions, n_features, eval_model, target_model):
self.params = {
'n_actions': n_actions,
'n_features': n_features,
'learning_rate': 0.01,
'reward_decay': 0.9,
'e_greedy': 0.9,
'replace_target_iter': 300,
'memory_size': 500,
'batch_size': 32,
'e_greedy_increment': None
}
# total learning step
self.learn_step_counter = 0
# initialize zero memory [s, a, r, s_]
self.epsilon = 0 if self.params['e_greedy_increment'] is not None else self.params['e_greedy']
self.memory = np.zeros((self.params['memory_size'], self.params['n_features'] * 2 + 2))
self.eval_model = eval_model
self.target_model = target_model
self.eval_model.compile(
optimizer=RMSprop(lr=self.params['learning_rate']),
loss='mse'
)
self.cost_his = []
def store_transition(self, s, a, r, s_):
if not hasattr(self, 'memory_counter'):
self.memory_counter = 0
transition = np.hstack((s, [a, r], s_))
# replace the old memory with new memory
index = self.memory_counter % self.params['memory_size']
self.memory[index, :] = transition
self.memory_counter += 1
def choose_action(self, observation):
# to have batch dimension when feed into tf placeholder
observation = observation[np.newaxis, :]
if np.random.uniform() < self.epsilon:
# forward feed the observation and get q value for every actions
actions_value = self.eval_model.predict(observation)
print(actions_value)
action = np.argmax(actions_value)
else:
action = np.random.randint(0, self.params['n_actions'])
return action
def learn(self):
# sample batch memory from all memory
if self.memory_counter > self.params['memory_size']:
sample_index = np.random.choice(self.params['memory_size'], size=self.params['batch_size'])
else:
sample_index = np.random.choice(self.memory_counter, size=self.params['batch_size'])
batch_memory = self.memory[sample_index, :]
q_next = self.target_model.predict(batch_memory[:, -self.params['n_features']:])
q_eval = self.eval_model.predict(batch_memory[:, :self.params['n_features']])
# change q_target w.r.t q_eval's action
q_target = q_eval.copy()
batch_index = np.arange(self.params['batch_size'], dtype=np.int32)
eval_act_index = batch_memory[:, self.params['n_features']].astype(int)
reward = batch_memory[:, self.params['n_features'] + 1]
q_target[batch_index, eval_act_index] = reward + self.params['reward_decay'] * np.max(q_next, axis=1)
# check to replace target parameters
if self.learn_step_counter % self.params['replace_target_iter'] == 0:
for eval_layer, target_layer in zip(self.eval_model.layers, self.target_model.layers):
target_layer.set_weights(eval_layer.get_weights())
print('\ntarget_params_replaced\n')
"""
For example in this batch I have 2 samples and 3 actions:
q_eval =
[[1, 2, 3],
[4, 5, 6]]
q_target = q_eval =
[[1, 2, 3],
[4, 5, 6]]
Then change q_target with the real q_target value w.r.t the q_eval's action.
For example in:
sample 0, I took action 0, and the max q_target value is -1;
sample 1, I took action 2, and the max q_target value is -2:
q_target =
[[-1, 2, 3],
[4, 5, -2]]
So the (q_target - q_eval) becomes:
[[(-1)-(1), 0, 0],
[0, 0, (-2)-(6)]]
We then backpropagate this error w.r.t the corresponding action to network,
leave other action as error=0 cause we didn't choose it.
"""
# train eval network
self.cost = self.eval_model.train_on_batch(batch_memory[:, :self.params['n_features']], q_target)
self.cost_his.append(self.cost)
# increasing epsilon
self.epsilon = self.epsilon + self.params['e_greedy_increment'] if self.epsilon < self.params['e_greedy'] \
else self.params['e_greedy']
self.learn_step_counter += 1
def plot_cost(self):
import matplotlib.pyplot as plt
plt.plot(np.arange(len(self.cost_his)), self.cost_his)
plt.ylabel('Cost')
plt.xlabel('training steps')
plt.show()
def run_maze():
step = 0
for episode in range(300):
# initial observation
observation = env.reset()
while True:
# fresh env
env.render()
# RL choose action based on observation
action = RL.choose_action(observation)
# RL take action and get next observation and reward
observation_, reward, done = env.step(action)
RL.store_transition(observation, action, reward, observation_)
if (step > 200) and (step % 5 == 0):
RL.learn()
# swap observation
observation = observation_
# break while loop when end of this episode
if done:
break
step += 1
# end of game
print('game over')
env.destroy()
if __name__ == "__main__":
# maze game
env = Maze()
eval_model = Eval_Model(num_actions=env.n_actions)
target_model = Target_Model(num_actions=env.n_actions)
RL = DeepQNetwork(env.n_actions, env.n_features, eval_model, target_model)
env.after(100, run_maze)
env.mainloop()
RL.plot_cost()
参考文献:
https://www.jianshu.com/p/10930c371cac
https://github.com/MorvanZhou/Reinforcement-learning-with-tensorflow
http://inoryy.com/post/tensorf
本文转载自 Alex-zhai 知乎账号。
原文链接:https://zhuanlan.zhihu.com/p/70009692
更多内容推荐
我的算法学习之路
一点儿经验,希望对想学算法的你有帮助
2021-01-29
深度学习基础入门篇 [四]:激活函数介绍:tanh、sigmoid、ReLU、PReLU、ELU、softplus、softmax、swish 等
深度学习基础入门篇[四]:激活函数介绍:tanh、sigmoid、ReLU、PReLU、ELU、softplus、softmax、swish等
2023-04-12
每个 Java 程序员都必须知道的四种负载均衡算法
一般来说,我们在设计系统的时候,为了系统的高扩展性,会尽可能的创建无状态的系统,这样我们就可以采用集群的方式部署,最终很方便的根据需要动态增减服务器数量。但是,要使系统具有更好的可扩展性,除了无状态设计之外,还要考虑采用什么负载均衡算法,本
2023-01-10
Redis 实现 feed 流
Feed 流中的每一条状态或者消息。比如朋友圈中的一个状态就是一个 Feed,微博中的一条微博就是一个 Feed。
2021-11-11
强化学习基础篇 [2]:SARSA、Q-learning 算法简介、应用举例、优缺点
SARSA(State-Action-Reward-State-Action)是一个学习马尔可夫决策过程策略的算法,通常应用于机器学习和强化学习学习领域中。它由Rummery 和 Niranjan在技术论文“Modified Connectionist Q-Learning(MCQL)” 中介绍了这个算法,并且由Rich Sutton在注脚
2023-06-02
11|地球往事:为什么 CV 领域首先引领预训练潮流?
在这节课中,我们来学习视觉预训练模型PTM。
2023-09-04
动手实践丨基于 ModelAtrs 使用 A2C 算法制作登月器着陆小游戏
在本案例中,我们将展示如何基于A2C算法,训练一个LunarLander小游戏。
2022-11-23
12|博观约取:重走 NLP 领域预训练模型的长征路
在这节课,我将带你解决自然语言处理领域所独有的一些问题,重走NLP“长征路”,看看 NLP 模型预训练技术在黎明前都经历过哪些考验。
2023-09-06
07|模型工程:算法三大门派,取众家之长为我所用
目前,以深度学习模型为代表的连接主义派表现出色。然而,在许多情况下,AI系统仍然需要结合其他两个派别的算法,才能发挥最大的功效。
2023-08-25
记录
时间来到2020年尾巴,突然看到2014年自己写的博客。那会在自我加强算法,还写了一些很简单的小游戏。那会找工作是写博客的主要原因,也不图很多人看到,是比较自私的目的。
2021-11-28
强化学习基础篇 [3]:DQN、Actor-Critic 详细讲解
在之前的内容中,我们讲解了Q-learning和Sarsa算法。在这两个算法中,需要用一个Q表格来记录不同状态动作对应的价值,即一个大小为 的二维数组。在一些简单的强化学习环境中,比如迷宫游戏中(图1a),迷宫大小为4*4,因此该游戏存在16个state;而悬崖问题(
2023-06-03
第四范式自动化推荐系统:搜索协同过滤中的交互函数
本文介绍第四范式研究组将自动化机器学习技术引入推荐系统中的一次尝试。
15|显微镜下的 Stable Diffusion(一):惊艳效果下的关键技术揭秘
这一讲我们会一起探讨SD模型的技术细节,包括文本引导原理、注意力机制原理、图生图的计算过程、反向描述词作用机制等。
2023-08-21
强化学习在美团“猜你喜欢”的实践
本文来自美团点评技术文章系列。
【牛客刷题 - 算法】NC7 买卖股票的最好时机 (一)
描述假设你有一个数组prices,长度为n,其中prices[i]是股票在第i天的价格,请根据这个价格数组,返回买卖股票能获得的最大收益 你可以买入一次股票和卖出一次股票,并非每天都可以买入或卖出一次,总共只能买入和卖出一次,且买入必须在卖出的前面的某一天
2022-10-03
1. 状态与状态空间
2023-09-26
强化学习入门——说到底研究的是如何学习
自机器学习重新火起来,深度强化学习就一直是科研的一大热点,也是最有可能实现通用人工智能的一个分支。
22|AI 图像编辑:如何用 Prompt2Prompt 实现“言出法随”
今天这一讲,我们将会学习“修图三部曲”:Prompt2Prompt、InstructPix2Pix和Null-Text Inversion这些指令级的修图技术。
2023-09-06
数据结构和算法学习总结 - 复杂度分析
数据结构和算法要解决的问题,1是快,2是省。 1.快,让代码运行的更快,代码具有更低的时间复杂度。 2.省,让代码更省存储空间,代码具有更低的空间复杂度。 复杂度分析是整个算法学习的精髓,一定要掌握好。
2021-01-31
什么是 Spring-Cloud、需要掌握哪些知识点,Java 面试常问的算法题
zuul:routes:consumer1: /FrancisQ1/**consumer2: /FrancisQ2/**
2021-09-11
推荐阅读
4. 启发式搜索:A* 算法
2023-09-27
DPO 直接偏好优化:跳过复杂的对抗学习,语言模型本来就会奖励算法
2023-07-13
强化学习从基础到进阶 - 案例与实践 [6]:演员 - 评论员算法(advantage actor-critic,A2C),异步 A2C、与生成对抗网络的联系等详解
强化学习从基础到进阶 - 常见问题和面试必知必答 [3]:表格型方法:Sarsa、Qlearning;蒙特卡洛策略、时序差分等以及 Qlearning 项目实战
2023-06-23
26|模型工程(二):算力受限,如何为“无米之炊”?
2023-10-18
大语言模型的预训练 [1]: 基本概念原理、神经网络的语言模型、Transformer 模型原理详解、Bert 模型原理介绍| 社区征文
2023-07-17
27|DALL-E 3 技术探秘(二):从 unCLIP 到缝合怪方案
2023-11-09
电子书
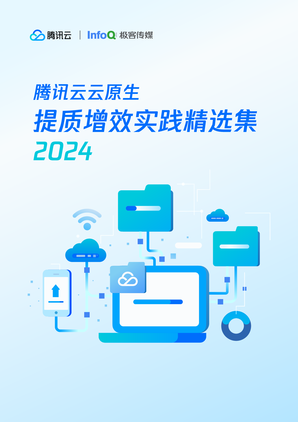
大厂实战PPT下载
换一换 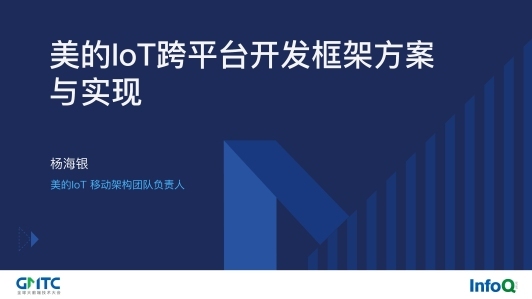
杨海银 | 美的 IoT 软件开发移动总架构师
王涛 | 字节跳动 APMPlus Android 负责人
王耀 | 百度智能云 技术委员会主席
评论